2025 GenAI Predictions for IT Insiders
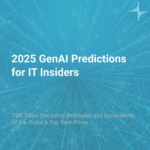
How are research firms, including TBR, and market and competitive intelligence groups within technology vendors using powerful new generative AI (GenAI) tools?
In this episode of TBR Talks, TBR Senior Vice President Dan Demers joins Patrick to discuss how global systems integrators, telecom vendors, hyperscalers and others are deploying GenAI internally to improve operations as well as how they are delivering GenAI solutions to enterprises across the global ecosystem.
Listen and learn with TBR Talks!
Submit your Key Intelligence Questions for Patrick and his guests
Connect with Patrick on LinkedIn
Connect with Dan on LinkedIn
Learn more about TBR at https://tbri.com/
TBR Talks is produced by Technology Business Research, Inc.
Edited by Haley Demers
Music by Burty Sounds via Pixabay
Art by Amanda Hamilton Sy
2025 GenAI Predictions for IT Insiders
TBR Talks Host Patrick Heffernan: Welcome to TBR Talks: Decoding Strategies and Ecosystems of the Globe’s Top Tech Firms, where we talk business model disruption in the broad technology ecosystem from management consultancies to systems integrators, hyperscalers to independent software vendors, telecom operators to network and infrastructure vendors and chip manufacturers to value added resellers. We’ll be answering some of the key intelligence questions we’ve heard from executives and business unit leaders among the leading professional IT services and telecom vendors.
I’m Patrick Heffernan, Principal Analyst, and today we’ll be talking about 2025 predictions for GenAI with Dan Demers, Senior Vice President of sales and marketing.
Evolving GenAI questions
Dan, thank you so much for being on the podcast. Always good to have a chat, I know we had a really good conversation last season. And what I want to start off with this season is talking about what’s changed around generative AI. And I want to ask you, particularly because of your role here at TBR, you talk to clients across the entire spectrum. So, consultancies, IT services companies, telecom vendors, infrastructure OEMs, cloud everybody. So, you hear their questions and I’m curious when you look back on the last year and think about generative AI, how have those questions changed?
Dan Demers, TBR Senior Vice President: Well, thanks for having me, Patrick. It’s a pleasure to be here. Yeah. Interesting question, the way that you frame it. So being one of the leading advocates for TBR out in the marketplace, I get to travel, I get to travel with you, other analysts, and I do speak with front line intelligence folks inside all those companies as well as executives. And I think you can go back about two, maybe two and a half years now where generative AI, ChatGPT everything really started to break. And we immediately got, I would classify it as kind of two sets of questions. Because TBR is known as a company that can dive into the operational performance of these technology companies, people come to us and ask for an operational breakdown. So, companies were asking us if we’re going to compete against Google, against Dell, against Microsoft, against pick the company, they’re asking us what is their customer zero approach to GenAI. So, we immediately began to get questions, what are you hearing about? And can you share anything about what are these companies doing internally? And simultaneous to that, as they were looking at internal adoption of agentic AI, generative AI, they were trying to understand what are the emerging use cases, what are people putting out there from an experimental, a developmental standpoint? What kinds of proofs of concept were people baking? And as soon as those conversations around those early stage deployments, we immediately started to get commissioned to do primary based research in looking at what are the paid POCs. It immediately- the entire explosion of the technology and the adoption of the technology was accelerated. And we were getting questions week after week of the entire acceleration of that adoption, as far as what are the OEMs doing for infrastructure here? What are the SIs doing for integration and digital transformation, and how are they using this with S/4Hana migration? All of the questions that we get around every other aspect of technology.
Patrick: Right.
Dan: You could almost guess that they were going to layer in so what does that mean for GenAI? What does that mean for artificial intelligence, machine learning, robotic process automation, how is that being implemented.
So, it really became two paths. What are people doing internally? How are they gaining efficiencies? I mean, heck, we had a long conversation at the beginning of last year about how the entire labor pyramid was going to collapse.
Patrick: Correct.
Dan: And how- and I think we’ve seen that
Patrick: Yes.
Dan: There’s been significant- your prediction was accurate. The reductions in force, the optimizations, whatever people want to call it. There were highly profitable companies that shed headcount in ways that, I’m sure for the folks who were unfortunately let go, didn’t make sense to them, but at a super high level inside those organizations, they had to stay in front of the next company on Wall Street to make hard cuts to optimize their labor pyramids to ensure that they were automating what could be automated, that they were on that bleeding edge of automation so that no one else could get in front of them.
Language models and TBR data
All of which leads me to my second question, because now we’re talking about language models, which 3 or 4 or 5 years ago, neither of us knew what a large language model or a small language model was. Now we talk about them all the time. And our clients are using our stuff in their models.
Dan: Yes.
Patrick: Can you talk a little bit about how that flip switched and then what, you know, what we’re going to see going forward in 2025 with that.
Dan: Well, I think it’s to the point about a discrete, defined small language model. We were approached, right after the wave of adoption of these ChatGPT-like tools by a handful of our customers, the majority but not all, the majority of them in the advisory, integration, professional services space. And they sought license to ingest in a, you know, a private environment in their own environment, our data, both our qualitative and quantitative, in a discrete small language model. And they wanted to begin to understand what licensure would look like, how they could access, what kinds of API feeds, what kinds of security protocols. And then we quickly began to explore and discuss the kinds of limitations of what a generative AI model could do with our proprietary intellectual property. Not knowing necessarily what our immediate peers were doing with these customers, but you can start to discern the kinds of questions we’re being asked and the ways in which they’re seeking to commercialize that engagement. Everyone recognized that is a value to that, and there’s very, very complex technologies, blockchain ledgers and, you know, credits and bits and pushes and pulls, versus an all you can eat, easy to process, easy to manage model.
So, we very early on, in one of the very first firms that started to do this internally, as they were building their own series of small language models, and without getting into anything confidential, proprietary, an array of models that in an appropriate way communicated in such a manner as to maintain the hygienic quality of each distinct model, but yet aggregate it up into a summarization tool and a generative tool in certain respects. And so, what we’re seeing is, again, we’re engaging commercially with a number of these advisory firms and other firms in the IT services space, in the IT infrastructure space, looking at how our- the key things that I remember them stating, we’re seeking well-architected, trusted, clean data, ideally propriety data. Anybody and everybody can crawl what’s available. And there’s, you know, various legal and ethical questions coming out on information that’s in the public domain, information that’s available publicly but isn’t public domain information. Versus a trusted source where they could enshrine licensed rights that their legal team was comfortable with. And so early on, we made as easy a tool to work with a these firms are able to a weekly basis, cache our entire live database. They can vet new data from old data. So, they have in their secure instance, of an SLM of TBR, and they’re able to crawl that with the appropriate licensure where we’re comfortable with how that’s established. And for us, we preferred it to be a simple model, versus these kinds of credits in blockchain ledgers and very advanced technology that in many ways duplicates the cost structure of public cloud. And I think we’ve seen the ease with which people can migrate workloads to public cloud, but then they get these bills and they’re scratching their heads saying, “I was told this would be so much cheaper.” So instead of trying to build this ever-spinning faster needle and meter of fee, TBR has decided early on let’s be the easy partner to work with. So, we do flat rate, it’s push pull as many times as you want, as many calls as you want, and we grant licenses so that our clients who have these tools, they can feel comfortable using our data, using our analysis as either a generative output of our SLM or as a combined output of an aggregation of other SLMs, and they’re able to use it internally, externally, to their heart’s content. And we’re seeing for TBR an absolute increase in the utilization of our data and analysis, because we’re seen as a vendor that’s not going to have that meter spinning and spinning and spinning. And so those responsible for the for the bill realize, well, at least we don’t have to gate or meet or restrict the TBR SLM in that case. So, it’s a really interesting I think, evolution.
things that they can find in the open market. And it’s shortcuts, it’s helping them get faster, it’s helping them be more creative. It’s decreasing the amount of time to build these sorts of mundane lists that oftentimes take hours, and, you know, these tools can do it in a minute. But it’s tapping into what’s publicly available. And what we’re realizing is, even if our analysts begin to explore how they can collect different public sources of information so that they have an easy place to work from, we get relied on because our analysis and our data is- the core is proprietary. You can’t get it publicly. And I know that’s what, when a firm that’s using us as, we are their vendor, they’re using internal data sources. They’re using, I can only imagine other third-party data providers, they’re using us. And it’s that understanding that, sure, this language model is out there, you know, cruising sesamestreet.com, you know, it’s getting information from every source.
Patrick: Right.
Dan: But then there’s that, as they said, trusted, clean, well-architected data that can’t be found anywhere else. So, I think that’s going to be a really strong place for enterprises to figure out how to play, for those advisory led firms who have the risk and compliance and the governance strength to be able to help design that. And then ultimately for data providers no longer being able to just, you know, artfully reproduce what’s publicly available, but to genuinely produce meaningful, proprietary, impossible to get elsewhere analysis and data that’s going to prove to be invaluable.
Patrick: From a trusted source, I think that’s the most important piece of it, because when I look at it, not from the TBR commercial terms or from the ease of doing business perspective, but from the how is TBR’s data going to be used in the real world? How is going to be used by our clients? What are the challenges that they’ve already run into that we could only make worse if we don’t do it right, but we can make better if we do it right. And because it’s a trusted source, and like you said, because it’s proprietary, they can’t get it from anywhere else, it’s coming to them as a trusted source. I keep coming back to that because the biggest stumbling block to faster adoption of generative AI solutions is data; is data orchestration, data cleansing, data management, and all of that. And so, when it’s coming from a trusted source, you immediately check all those boxes right away. And so not only is it the easier to work with commercial terms, it’s also the data that’s trusted from a trusted source. And that makes such a huge difference.
Dan: Absolutely
Use cases
Patrick: I’m curious too. So, the other thing, not only do you hear questions, but you do hear use cases. You do hear some wild stories about- well, I’m guessing some of them are wild, about what clients are actually doing out there. What some of the GenAI sort of, “here’s a crazy story, can you imagine we were able to do this?” Are there any that kind of come to mind? I feel like at the beginning of generative AI, there were all kinds of, you know, use cases around how we got rid of half of our marketing department because we were able to create collateral just using, you know, ChatGPT and Copilot. But we’ve passed that now. We’re past that first stretch of crazy stories. Are there any that you’ve heard recently or that you think maybe are going to be coming in the near the near future? Use cases that are really centered on deploying generative AI in a way that’s creative or a way that’s new.
Dan: I don’t know about creative, but the one that I hear time and time again from your colleague in the telco space, Chris Antlitz and his staff are talking a lot about call center disruption, and I have not sat in on any briefings or calls with either our telco vendors or telco operators to really hear that play out. But as a consumer, especially as someone who you know from time to time, you have to interact with like a doctor’s office or a hospital or an auto dealer or- I’m dealing with call centers as a consumer, and that experience continues to decline in user quality. It’s horrible. And there are times when, although today you really can’t tell if it’s an artificial-intelligence-backed bot speaking to you, it’s become harder and harder too. You know, 2 or 3 years ago you could, and getting prompted to assert or state what it is you want or need and it’ll be great when that actually works, because I don’t think that’s working yet.
But that’s one of those promises to come that I’ve heard a lot about in the last 6, 12 months. That disruption to the call center and how that can increase customer satisfaction and retention, I’ve heard those assertions made, if that’s a promise they could keep, I think you’d end up with a lot of happy customers. And I think you could really have an impact in your business if that truly would work.
Patrick: Right. If you get the efficiency and especially if you get the customer retention.
Dan: Yeah.
Patrick: Because I think that’s one thing we have seen with generative AI is that it has been that accelerating technology, which is pulling through robotic process automation, pulling through AI generally, pulling through it at through analytics. Eventually, you know, knock on wood here, it’s going to pull through blockchain. I mean, there’s a lot of technologies that are going to come with it. And when those technologies are also adopted at a more accelerated pace, you’re going to see the disruption across businesses. I mean that’s the history of businesses, that when new technologies come along, that you get new entrants into an ecosystem that disrupt everything. So, we’re going to see companies that are going to need to retain their clients and be more efficient and call centers are one area where that can make a big difference.
Dan: The other the other thing I’ve seen personally, so as a sales executive, I receive quite a bit of unsolicited inbound inquiry from other salespeople trying to sell a solution to me. And inevitably, just with social media tools being the way they are, I have in my algorithm an endless array of 15 second and 30 second videos on AI tools for salespeople. And one of the things I recall seeing in the last couple of weeks was a chat bot that was engaged by another chat bot, and it was an AI clearinghouse of sales tools, and they were showcasing how the bots have gotten such a place where they can fool one another. And it was a sales back and forth between customer-bot and sales-bot that as someone who’s sat through enough sales trainings in my life and I’ve led plenty over the years, it looked like the best role play I’ve ever seen. And it was just two chat bots literally going back and forth.
Patrick: Right.
TBR use cases
Dan: So, I certainly can see, and we’ve been approached as an organization with the new tools that we’re launching in terms of our client portal, bringing a bot to life. You know, we’ve joked about probably one of our top three analysts, he’s on your team, Boz. Boz Hristov, you know, we joke about having a Boz-bot.
Patrick: Yeah.
Dan: And woe be the day when that ever happens, because the world will bow at the Boz-bot’s feet.
Patrick: *laughs* Right, yes.
Dan: But to be able to create, I think that’s the promise, right? If we can have a tool that can really read and map all of our intelligence, our analysis, our data, to be able to posit solutions to the search queries that can then point back to the actual research itself and annotate and provide citation to different data sets into different research streams. For us that’s sort of that next evolution because while we have a discrete and finite pool of data, as I know I’ve heard you say, you’ve said this many times, it’s a relentless stream of data.
Patrick: Yes.
Dan: So, our entire data lake of quant and qual is essentially refreshed every three months. And it’s voluminous. And to be able to track that and to be able to extract what you want out of that and what you need out of that, the new tool does that to a good deal. But to be able to have a generative tool that can help stitch together answers and inputs and ideas across various analysts’ outputs. I think that, for us, becomes sort of that next step that I’m eager to see us take in the coming year.
Patrick: Yeah, I was going to wrap up by asking you where you think we’re going with all this, but that really summed it up nicely.
I do think the- I have always talked about how we relentlessly produce, we also relentlessly research. And so, we’re going to reach an inflection point hopefully again, knock on wood, where that Boz-bot in reality is simply being able to tap into everything he’s researching all the time. And so, you won’t need to wait for Boz to write a report. You’ll be able to tap into the research he’s doing now, what he’s thinking, how he’s reacting to what he’s reading relentlessly. It’s frightening. Yet it might be coming soon.
Dan: You know, and I think we’ve already heard from users. So among our tens and tens of thousands of users every week, every month, there are people that we can see will download Catie Merrill’s research consistently, or Boz’s research religiously or Chris Antlitz or Mike Soper, etc. so we know that there are people who, because of their needs, their workflows and their interests, they’re reading everything that, Steve Vachon is writing.
Patrick: Right.
Dan: And so, if we can generate a tool that helps Steve amass and organize everything that he has on his weekly monthly reading list, and his listening list, and all of a sudden you’ve got a transcript of every single investor call that he sits in on, if he has all of the reading materials. And now all of that is in his little Vachon-bot or Boz-bot, and then it’s not necessarily going to take his place. It never will. You can never replace Catie Merrill, but there’s always going to be that bandwidth limitation. Right. And I know Catie’s value is briefing and speaking to companies like Accenture or Informatica or Dell or whomever. The highest value that she can bring, or Steve can bring is that on the fly, lifetime of knowledge of what these companies do, how they run, their corporate culture strategy. You can make assertions, and you can help our clients project and predict future behaviors and partnership dynamics. And then all that just hard labor of consuming if we can, if we can help them shortcut that. It’s still their trusted sources; it’s still their data. They’re getting dirty in their data. But we can just help augment that. They’ll be able to spend more time delivering the real value, which is you’ve sat in on these calls just as I have. You’re more of a generalist, and you’ll bring in the expert, you’ll bring in Catie Merrell, and she’ll talk about the deep knowledge she has on Equinix as an example.
And Equinix. Right,
Patrick: Right.
Dan: They’re a critical component of the ecosystem. And who has time to master every company in every dimension of this ecosystem.
Patrick: Right.
Dan: Well, good thing we’ve got a team of experts who focus on a discreet 3 to 7 companies, and they can tell you anything that you need to know about, how does Digital Realty partner with HPE versus Dell, and what’s the advantage and who has the better relationship, and what does that mean and which metro area? I’m clicking into data center colos. But that’s just that’s just one- that’s one orientation of a flavor of expertise that our team brings. And to be able to free her up, to be able to speak more to the client, to speak more to the market. I think that’s the ultimate promise to be able to take what’s public, to be able to build our proprietary, and then to be able to speak that out. That’s where I see AI at TBR, and I can only imagine our peers and friends at places like IDC, Gartner, etc. are all doing the same kind of thing.
Patrick: Yeah.
Dan: How do how do we free up our experts to deliver the highest value? How do we give them tools that they themselves trust? Because they’re the ones that have to defend the analysis, defend the data; they need to rely on the trusted workability of that tool so that they can be out there helping our clients be time to decision, make the right investment calls, make the right partnership calls, make the right staffing decisions for the right partnership in the right geo in the right industry vertical.
Patrick: Right.
Dan: That’s our gold. That’s our specialization. And the more we can do that for clients, either on a Teams call or face to face, it gets back to the trusted source of data. Right. And how do we get to that trusted source of data to become proprietary so that no one else has it, and we know how to deliver it. And the Steve Vachons of the world and the Catie Merrills of the world know how to deliver it.
Patrick: Yeah. I know we’re going long here, but I’ve got to bring this up because you sort of hinted at it, you’re sort of going down a road. That reminds me of when I first started doing competitive intelligence with Deloitte way back in the day. And one of the first lessons I got from a guy named John Shumadine, at the time with Deloitte, not there anymore, but anyway, was in order to be really good at providing competitive intelligence, I had to know what my readers were reading. It wasn’t enough to go to them with what I thought was important. I had to know, what are they reading every day? So, you know, is the head of consulting for Deloitte, reading the Wall Street Journal or the Financial Times or the New York Times on his car ride in in the morning, like what’s his paper? What’s his reading? Because you didn’t want to give them stuff they already knew because they had already read it. What you started to hint at was this idea of our clients being able to understand, because they’re seeing all the data that we’re using because we’re a trusted source, the Boz-bot or the Vachon-bot, well they’ll eventually know where are all the things that we’re pulling from. What are the things, like I said before, like being able to tap into that relentless stream of research. So, building up that sense of trust. And it’s a weird idea to think, okay, our clients are going to have to know what is Boz reading, what is Boz thinking, where is Boz is getting his information. But that’s actually going to be very valuable for them in terms of building up that trust and knowing that, it’s a way of taking it from this is a valuable source of information, to this is a valuable, trusted, relentless, I know where it’s coming from, and why it’s giving it to me, and why it’s seeing it that way kind of source and that that, I think, is where we go from just being able to provide what you talked about, that ability to make better, quicker, faster decisions, to the confidence behind being able to make those decisions because you got this, you got TBR behind you basically.
Dan: Yeah, we know we have a very interested potential new partner, new client when they start asking those questions, help me understand how is Boz getting at this? Show me Alex Demeule’s methodologies and how is he arriving at-? Tell me again how you’re able to get Adobe’s trailing 12-month revenue by partner? How do you know Accenture and Adobe’s breadth and depth of their-? It’s not behind closed doors, but it’s our ability to unpack how Salesforce, Adobe, SAP, Azure, how they’re partnering with the top 17 or so global systems integrators to drive the majority of enterprise spend.
Patrick: Yes, yes.
Dan: It’s growing. This is an ever growing more important aspect of the market. And our team of experts have unpacked kind of the secret code to building out revenue credentialing, training, headcount, build with, design with, sell with. “How is that possible?” And once they understand and they can see the math and they can see the assumptions and they can understand how we’re sourcing this, well then this whole new world of decisions that can be made based on objective data opens up to them.
Patrick: Right.
Dan: Whereas before. “Well, I know Accenture.”
Patrick: “I got a guy.”
Dan: Yeah, “I got a guy. He’s good.” And you know what he is good.
Patrick: Yeah.
Dan: We know the guy too. He’s a great guy. You’re going to go have dinner with him in Dallas.
But it’s not about knowing the guy. It’s about, what is that guy? And then what is his immediate peer at, say, Deloitte or at, say, EY, KPMG, etc.? What do all of them do with SAP?
Patrick: Right.
Dan: Versus Oracle versus Workday versus ServiceNow versus Dell.
Patrick: Right.
Dan: Those are the questions that- and it comes back to what are they reading, what’s the data they’re compiling, how are they- you know the combination of cross practice, internal TBR talk right, bringing data from two different teams that ten years ago, really almost never spoke to each other.
Patrick: Yeah.
Dan: It’s just the workflow thing. Everybody liked one another. It was just people had models sitting on their own private machines, and the digital transformation of TBR has been- I mean, again it goes back to customer zero, right? We’re preaching this, we’re practicing this, and we’re seeing the benefits of this as I think finally, good data is being implemented, good architecture, trusted sources. I think enterprise is really going to start to unpack that value. It’s probably been a little bit longer in terms of enterprises expectations versus reality, maybe a little bit like this podcast, a little bit longer than perhaps I thought.
Patrick: That’s all right.
Final thoughts
Dan: But, you know, to put a bow on it, I think the value is going to start to get realized, that the revenue is going to go up the chain a little bit, based on what I hear you all talk about. I think the same for us. We spent a lot of money on digital transformation, we’re seeing massive dividends, last year, this year with new tools, highly proprietary, actionable intelligence. And we’re looking at AI tools ourselves and how that’s going to help our client and our user experience really explode value each individual user and each client.
Patrick: And we’re setting an example, we’re customer zero for breaking down silos and you can make things actually happen.
Dan: And it worked. It’s fantastic. Yeah.
Patrick: Excellent Dan, thank you so much. We will reconvene in a few months and have another chat.
Dan: Love it. Thanks a lot.
Patrick: Thanks.
Next week, I’ll be speaking with TBR Senior Analyst Ben Carbonneau and Research Analyst Alex Maxfield about TBR’s 2025 predictions for AI PCs. Don’t forget to send us your key intelligence questions on business strategy, ecosystems, and management consulting through the form in the show notes below. Visit tbri.com to learn how we help tech companies large and small, answer these questions with the research, data, and analysis my guests bring to this conversation every week.
Once again, I’m your host, Patrick Heffernan, Principal Analyst at TBR. Thanks for joining us and see you next week.
TBR Talks: Decoding Strategies and Ecosystems of the Globe’s Top Tech Firms
Join TBR Principal Analyst Patrick Heffernan weekly for conversations on disruptions in the broader technology ecosystem and answers to key intelligence questions TBR analysts hear from executives and business unit leaders among top IT professional services firms, IT vendors, and telecom vendors and operators.
“TBR Talks” is available on all major podcast platforms. Subscribe today!